All published articles of this journal are available on ScienceDirect.
Factors of Schedule and Cost Performance of Tunnel Construction Megaprojects
Abstract
Aims:
This study illustrates the main factors that influence the cost overruns and schedule delays of tunnel construction megaprojects.
Objective:
An empirical analysis was carried out based on a dataset collected from a number of recent tunnel megaprojects worldwide.
Methods:
Analyses of variances and regression analyses were conducted to infer statistical significance and understand the relationships that exist between cost overruns, time delays and variables of context, technical, and governance characteristics of the sample projects.
Results:
The most significant factors are those related to the complexity of infrastructure and the type of contracting system used to deliver the project. In particular, some technical characteristics pertinent to the size of the tunnel reveal to be influencing factors of both schedule delay and cost overrun, while the usage of a traditional contracting mechanism is likely to impact the cost overrun. The type of infrastructure, region, ownership, and funding scheme are not found to be statistically significant determinants of cost and time performance.
Conclusion:
This analysis reaffirms that the size and complexity are important factors of typical low performance of tunnel construction megaprojects. The results of this study can be used for strategic design and planning by decision-makers, project managers and designers.
1. INTRODUCTION
Megaprojects often fail to meet the predetermined targets of cost and time performance [1-3]. Megaprojects are characterized by design complexity, the number of stake- holders involved, huge budgets, long durations and major uncertainties, so a small change in the project input may reflect in a large and escalating effect in the project output and poor delivery both in terms of cost and schedule performance [4, 5].
These factors of poor performance that are observed in large-sized projects also apply to tunnel transport megaprojects, which are reported to experience substantial cost overruns and schedule delays [6-8].
However, despite the availability of studies on construction megaprojects and associated cost and schedule performance [9-11], little research is available about the cost and schedule performance of tunnel transport megaprojects [12] and the research works that specifically focus on understanding the factors of cost overruns and time delays are just a few [13]. These few available studies reinforce the need for investigating the problem of the low cost and schedule performance that is experienced by most tunnel transport megaprojects.
To fill this research gap, this study performs an empirical analysis of the cost and schedule performance of a sample of tunnel megaprojects excavated worldwide in order to understand the main factors that may influence cost overrun and schedule delay of tunnel megaprojects in transport infrastructures. In particular, the analysis investigates project, technical and governance characteristics that are likely to cause low schedule and cost performance recorded for most tunnel transport megaprojects.
This is done in an attempt to help improve the cost and time performance of future tunneling projects and establish better organizational and managerial policies when dealing with such transport infrastructure megaprojects.
To this end, the paper is structured as follows. First, we review the available literature with a focus on previous empirical studies about the typical low performance of megaprojects and mega-tunnels. Second, we illustrate the empirical research methodology and present the dataset. Then, we analyze and discuss results. Finally, we give the main implications, draft future research directions, and draw the conclusion.
1.1. Previous Studies
Recent studies show that megaprojects in the construction sector often fail to meet original cost estimates, baseline schedules and other projected outcomes [14]. As a conse- quence, the high failure rate experienced by such projects has originated several studies to examine their key success factors [15, 16].
Previous works have placed emphasis on project cost esca- lation, poor estimation, complexity, and risk factors [17-20].
With this regard, there is a founding empirical study to understand the factors of typical poor performance in transport megaprojects [21]. Based on the regression analysis of a sample of 258 infrastructure projects from 20 countries and worth around 90 billion US dollars, the authors report that cost overrun and time delay are a widespread global reality, regardless of the historical period, region, or mode of transport. Also, cost overruns are reported to depend on three main factors: the duration of the construction phase, the size of the project, and the type of ownership [22].
More recently, researchers have started to widen the scope of possible failure factors and have focused more on the structural characteristics of the project context and its impact on project success. According to a study by Asvadurov et al. [23], infrastructure megaprojects with a budgeted cost greater than one billion US dollars are delayed by one year and exceed the expected budget of over 30%, on average. Specifically, that study found that only 5% were completed in line with the expected duration and budget. Instead, the average cost overrun is 37% for all kinds of projects, while for transport infrastructure, it raises to 40%. Love et al. [24], present an empirical study to examine the cost performance of public infrastructure projects delivered from 2011 to 2014. Out of this analysis, cost performance ranges considerably, with a mean cost overrun of 23.75%. No significant difference in cost overrun is found in relation to the project’s contract value, types and procurement method, while change orders account for a significant portion of the original contract value.
On the other side, Ardeshir and Amiri [25], by applying conventional project risk analysis and analytical hierarchy process approaches, find that scope changes in water conveyance tunneling projects account for considerable changes in original cost, duration, safety and quality measures. To understand different risk factors that influence cost overruns, schedule delays and quality of project delivery, Muya et al. [26], conducted a survey among construction industry professionals in Zambia. They found the four groups of risk factors (financial planning and management, change manage- ment, lack of capacity and poor schedule management) that cause low performance in the delivery of construction projects. Also, Shane et al. [17], give a categorization of 18 primary factors of cost escalation of all types of construction megaprojects. In this stream of research, Cantarelli et al. [27], and Huo et al. [28], consider three technical variables, namely the project type, estimated budget and length of the project implementation phase as independent explanatory variables of cost overrun in transport megaprojects.
Another key success factor that has been receiving attention by researchers is the governance of megaprojects [29]. This stream of literature identifies the structural characteristics needed for a successful project execution. Project governance is about the use of delivery systems, authority and processes to control a project with the aim of supporting projects to achieve their cost and time objectives [30]. With this regard, some authors have been seeking to explain the significant performance problems incurred by many infrastructure and transport megaprojects and critically examining their governance solutions [31]. For example, Pinheiro Catalão et al. [11], incorporate exogenous, political, legal, regulatory and economic determinants of cost deviations in transport projects. To determine and understand various factors that cause delays in power transmission infrastructure projects, Pall et al. [32], analyzed 46 idiosyncratic delay indicators grouped into such classes as sector-specific, general, administrative, stakeholder, equipment and material, and external. They conclude, based on the results of multiple regression modeling and factor analysis of 311 practitioners’ response data, that the sector-specific (dead end towers, route changes, road construction, logistics) and general (poor communication between project parties, delays in payments, poor planning and scheduling, poor site management) factors are the most critical in such infrastructure projects.
However, despite the availability of studies that report about the schedule and cost misperformance of megaprojects, little research is available to explore the potential factors of cost overrun and schedule delay in tunnel transport megaprojects specifically. In fact, some studies focus on cost estimation models at the design phase of tunneling projects and analyze the main design factors to be considered [33, 34], depict how several variables affect the estimated original cost [35], and develop risk models to avoid duration and cost underestimations [36]. Also, some studies anticipate that management and control of risk are important factors to mitigate cost escalation in tunneling projects [37]. But only a very few pieces of research investigate the cost and schedule performance of the execution of tunnel projects, such as the case-based work by Han et al. [38], or the empirical research by Hilar and Srb [39], who analyze the technical factors of rail tunnels that have a marginal effect on the final cost of the tunnel infrastructure.
This paper fills this gap and provides an analysis of the determinants of poor schedule and cost performance of tunnel megaprojects. It is intended to complement similar works that have explored the cost overrun and schedule delay factors for megaprojects in general.
2. RESEARCH METHODOLOGY
The research methodology starts with the data collection in two stages; data collected from secondary sources and primary data collection for reconfirmation of the secondary data and completing missing information from the first stage. Then, the descriptive statistics are presented to provide an overview of the sample projects and reveal project characteristics (variables). Finally, the explanatory analysis using linear regression and associated statistical tests on the collected variables is presented to infer a possible impact of the project independent variables on the dependent schedule and cost variables.
2.1. Data Collection and Grouping of Explanatory Variables
In the first stage, potential tunnel transport megaprojects are identified via collecting relevant second-hand information through searches in web-based project databases, open data sources, scholarly publications, official websites released by either governmental agencies, project promoters or general contractor, as well as in available studies from the World Tunnel Congress 2017 (9-15 June 2017; wtc2017.com). Data are collected for tunnel construction projects in all rail and road modes of transport that have been completed internationally over the last 20 years with a budgeted cost greater than US$500 million. The selected threshold reflects the definitions of megaprojects as discussed in the literature by Invernizzi et al. [40] and Hwang [16]. The first dataset of 54 tunnel megaprojects is prepared based on a number of variables that are expected to impact the typical cost overrun and schedule delays of tunnel megaprojects. To measure such impact, both independent and dependent variables are collected at this stage. Independent variables include the name of the project, a short qualitative description, the countries crossed by the infrastructure, the mode of transport, the duration of the concession period, technical data about the tunnel topography, the original estimated duration and budget, the length of the infrastructure, the number of tubes and cross passage tubes, the excavation technique and governance information related to project owners, sponsors and contractors, delivery system used and the funding scheme. The indicators of cost overrun and schedule delays are also collected as the dependent variables of the problem under investigation. Here, cost overrun is defined as the percent final cost increase over the original budgeted cost. Similarly, the schedule delay is measured as the percent delay compared to the original duration.
The above explanatory variables are selected as supposed to be likely factors of schedule and cost performance based on either assumptions or previous literature evidence [41]. Based on this, the independent variables are further grouped into three categories: project, technical, and governance, which are analyzed in detail in the next section Descriptive Analysis of Dataset.
The basic hypothesis behind the analysis is that the greater the complexity of the infrastructure, in terms of size, design, technical characteristics and governance systems, the higher the risk and associated likely schedule delay and cost overrun for the project [19]. For instance, the scope, the scheduled duration of the construction works and the amount of capital expenditure required are typical determinants of greater scope creep, longer schedule slippage and higher cost overrun in a project. Similarly, the technical characteristics of the soil or excavation technique used and the type of delivery system may lead to diverse schedule and cost performance outcomes [27, 28].
The work follows with the second stage of the data collection since data from secondary sources of information were not available in all projects and for all variables. This originates the need for a direct primary data collection to fill in the missing information and double-check the consistency and reliability of the collected dataset from the first stage.
Accordingly, first-hand data are collected via preparing a questionnaire and inviting the project promoters/sponsors of the identified tunnel megaprojects to fill it online. The questionnaire is composed of 50 questions, subsumed into three sections corresponding to the defined project, technical and governance category of the variables. The project-related variables are the same as the secondary data collection phase and include the final cost overrun and schedule delay measured as per the above definitions of percent variations compared to the original estimated budget and duration, respectively. Also, the technical and governance group of the variables are the same as the ones collected in the first stage. The complete questionnaire can be obtained by contacting the corresponding author.
Invitations are sent directly by TELT Tunnel Euralpin Lyon Turin, public promoter of the alpine base tunnel from Turin to Lyon and the main sponsor of this study, to their peer entities acting as project sponsors of the tunnel megaprojects included in the dataset. The surveyed entities are identified by the public relations office of TELT. It is requested that the questionnaire is filled by the entities themselves with the help of their managerial and technical staff. One survey is sent out for each one of the projects considered in the secondary-hand research so that 54 surveys are sent.
Then, the first dataset is crosschecked with data obtained from the respondents, which are used as the primary data source when small discrepancies are recorded. When major discrepancies are observed, incomplete and/or inconsistent questionnaires are returned to the project promoters who are invited to reconfirm their data or revise with more accurate information.
Out of 54 surveys submitted, only 42 project question- naires are returned, with a response rate equaling 78%. Eventually, the sample of 39 projects with complete and consistent data from both secondary data collection and first-hand surveys are considered for further descriptive statistics and explanatory analysis using linear regression and associated statistical tests.
The sample includes the following projects: the Sydney Metro Northwest and Southwest&City tunnels in Australia, the Lainzer, Wienerwald, Semmering and Koralm tunnels in Austria, the Agua Negra Tunnel crossing Chile and Argentina, the West Qinling tunnel in the Gansu province of China, the New Guanjiao tunnel in the Chinese province of Qinghai, the Central-Wan Chai Bypass tunnel in Hong Kong, the Toyo and La Linea tunnels in Colombia, the Yulhyeon tunnel in South Corea, the Cityringen metro line in Denmark, the FinEst tunnel link from Finland to Estonia, the Bossler tunnel in Germany, the Fehmarnbelt Link connecting Germany and Denmark, the Seikan tunnel in Japan, the Jaipur Metro Phase 1 and Phase 2 in India, the Italian Terzo Valico, the TELT Moncenisio base tunnel from France and Italy, the Brenner alpine base tunnel from Austria to Italy, the Norvegian projects of Follo Line, Ryfast, Rogfast, and New Ulriken tunnels, the Metro Doha Phase 1 in Qatar, the Guadarrama tunnel in Spain, the Stockholm bypass tunnel in Sweden, the Ceneri, Lötschberg and Gotthard base tunnels in Switzerland, the Eurasia tunnel and the Üsküdar Ümraniye Çekmeköy metro project in Turkey, the Channel tunnel from the United Kingdom to France, and the Qamchiq tunnel in Uzbekistan.
2.2. Approach for Explanatory Analysis
After the data collection, we conduct an exploratory analysis to capture the main characteristics of the dataset. Then, an inferential analysis is developed to detect possible relationships between variables and, in particular, to analyze whether the project, technical and governance group of the factors may be having a statistical relationship with project cost and schedule performance. As already defined above, for the purpose of this study, schedule performance is measured as the percent variation, that is, the fraction of actual duration minus the scheduled duration over the scheduled duration. As for cost performance percent variation, this is measured as the actual final cost minus the original budgeted cost over the budgeted cost.
With this regard, to determine whether their differences were statistically significant, both one-way ANOVA and simple linear regression methods are used. The null hypothesis of the model is that the means of the comparable samples should be the same: for this hypothesis to be rejected, the p-value must be less than 0.1. If it is below 0.01, the difference is strongly significant; between 0.01 and 0.05, significant; between 0.05 and 0.1 weakly significant.
In particular, in order to verify the assumption of homoscedasticity, which is referred to as the homogeneity of the variances within the layers, a Levene test is used. Levene’s test null hypothesis is that the variances are equal within the layers: if this shows significance lower than 0.05, the interpretation is that the variances are not homogeneous and it is necessary to proceed with other tests than ANOVA to verify the equality of the means. To test the ANOVA assumption, which is the normality of the dependent variable within the different groups, the Kolmogorov-Smirnov test is used. Its null hypothesis is that the distribution is normal. The study tests both assumptions using the SPSS® software.
When both assumptions of the ANOVA model are violated, we have to use a Mann-Whitney's non-parametric test, extending to a Kruskal-Wallis test for those cases with more than two groups [42].
Due to the limited number of available observations, results obtained cannot be declared of complete statistical significance. However, the aim of this study is to detect the possible reasons and provide explanations for delays and cost overrun of large tunnel construction projects rather than to assert exact matches. The limited number of projects is inherently related to the worldwide arena of large tunnel infrastructures: it is intentionally decided to analyze megaprojects only, which are limited in number per se.
2.3. Descriptive Analysis of Dataset
The tunnel project characteristics in the dataset are subsumed and analyzed by the project-related, technical and governance-related group of variables.
Regarding the project-related variables, out of 39 tunnel projects, 7 are still in their design and planning stage, 19 are in progress and 13 have been completed. Eighteen were announced earlier than the year 2000, 16 from the year 2000 to 2010, and 5 after 2010. 13 projects were longer than 10 years, 20 projects lasted from 5 to 10 years, and just 6 were shorter than 5 years. Here, the duration is defined as the period of time from the date the project was publicly announced by the promoting government to the start date of traffic operations. The size of capital expenditure ranges from €0.5 billion to more than €4 billion, with 19 projects less than €2 billion, 6 from €2 billion to €4 billion, and 14 larger than €4 billion.
Nineteen tunnels are railroad infrastructures only, nine are used for road vehicles, 3 are for mixed usage and 8 metro lines. One tunnel is dedicated to freight transport only, 10 projects are for people transport, while the remaining 28 have mixed use. Twenty-three projects are alpine base tunnels, ten projects are urban infrastructures and 6 are in other non-alpine landscape settings. 23% of projects are transnational tunnels.
The dataset includes 56% projects developed in Europe, 28% in Asia, 3% in North America, 8% in South America, and 5% in Australia. Table 1 illustrates the main project-related characteristics of the sample projects. The average cost overrun and schedule delay of the sample projects are 27% and 34%, respectively. This is consistent with Flyvbjerg et al. [21], who recorded an average cost overrun of 34% for both tunnel and bridge projects. In particular, the average time delay is approximately 1.6 years.
Variable | Unit | Min | Max | Mean | St. dev. |
---|---|---|---|---|---|
Cost overrun | % | -3% | 132% | 27% | 31% |
Schedule delay | % | 0% | 100% | 34% | 29% |
Scheduled duration | years | 2 | 17 | 7.1 | 4.01 |
Approval period | years | 0 | 25 | 7.83 | 7.5 |
Excavation phase delay | years | 0 | 6 | 1.29 | 1.56 |
Budgeted cost | billions € | 0.5 | 9.61 | 3.37 | 3.01 |
Capex per km | mln €/km | 10.2 | 176.6 | 53.2 | 32.70 |
Variable | Unit | Min | Max | Mean | St. dev. |
---|---|---|---|---|---|
Infrastructure length | km | 5 | 135 | 52.4 | 39.3 |
Tunnel length | km | 2.8 | 85 | 24.6 | 18.9 |
Total length of tunnel tubes | km | 4.5 | 230 | 57.2 | 53.4 |
The internal diameter of single tube | m | 5 | 16 | 8.4 | 2.3 |
Distance between tubes | m | 0 | 40 | 29.3 | 13.0 |
Maximum altitude | m | -390 | 4080 | 583.7 | 1041.2 |
Maximum slope | ‰ | 3 | 72 | 21.7 | 17.8 |
Number of cross passage tubes | units | 4 | 268 | 81 | 64.1 |
Distance b/w cross passages | m | 100 | 3144 | 461.4 | 543.3 |
Tunnel Boring Machine (TBM) excavation portion | % | 0 | 100 | 65 | 35 |
Number of TMB used | units | 1 | 21 | 4 | 4.5 |
TBM length | m | 85 | 450 | 170.5 | 102.9 |
TBM weight | ton | 100 | 3400 | 1685 | 986 |
TBM diameter | m | 5.8 | 13.7 | 9.2 | 2 |
TBM average speed | m/day | 8 | 26 | 16.75 | 6.32 |
Traditional excavation speed | m/day | 3.7 | 6 | 4.7 | 1 |
Excavated rock volume | mln m3 | 0.1 | 19 | 6.6 | 6.3 |
Excavated rock weight | mln tons | 4 | 40 | 17.7 | 13.4 |
The average approval period, referred to as the preliminary time period before the project is initiated, from the date the project is publicly announced to the date it is formally authorized is 7.83 years with a high standard deviation: it took more than 25 years for some projects to initiate the construction phase.
Second, Table 2 reports the main technical variables of the sample megaprojects that may be impacting their cost and schedule performance. These technical variables can be subsumed into two main categories of factors that are expected to influence the project performance, namely: the dimensions of the project and the type of excavation. The dimensions of a tunnel megaproject may be in turn characterized by several performance variables, namely: the ‘Infrastructure length’, which is the extent of the entire road or rail infrastructure the tunnel is part of, the ‘Tunnel length’ itself, measured from end to end of the underground passageway, the ‘Total length of tunnel tubes’ which usually equals the ‘Tunnel length’ times the number of tubes the tunnel is composed of, the ‘Internal diameter of a single tube’ and the ‘Distance between tubes’ as main sectional factors, as well as the ‘Number of cross passage tubes’ and the ‘Distance between cross passages’ as longitudinal performance factors. Also, the ‘Maximum altitude’ and ‘Maximum slope’ are expected to be likely factors of cost and schedule performance as these may increase the construction risk in a tunnel project. As far as the excavation variables are concerned, the ‘Tunnel Boring Machine (TBM) excavation portion’ and the ‘Number of TMBs used’ are indicators of the level of construction automation, which is expected to reduce construction contingencies due to better technical control. However, larger TBM dimensions, namely: the length, weight and diameter, as well as higher TBM speed, may counteract and restrain the benefits of automated excavation. Similarly, the ‘Traditional excavation speed’ is measured together with ‘Excavated rock volume’ and ‘Excavated rock weight’ to understand the possible relations with cost and schedule performance when there is a larger portion of blasting excavation [43].
Finally, several variables related to governance issues are important factors of project performance. To analyze whether these aspects may influence the project schedule and cost performance, the governance variables are project delivery system, financing mechanism, payment scheme, and Opera- tions&Maintenance (O&M) service.
51% of projects are directly delivered by the national ministry of infrastructure or public agency using traditional contracting mechanisms; for 20% of the projects, a state or publicly-owned project company is created; the remaining 19% of projects are developed by a privately-financed project company shared by construction companies, service operators and other equity investors [44]. The Chinese projects belong to this last category, although the shareholders are government-owned. In summary, 39% of projects are developed by a Special Purpose Vehicle (SPV) that acts either as a Design-Builder (DB) (21%) or as a concessionaire responsible for all project lifecycle stages of design, build and O&M (18%). The concessionaire SPV is either public (10%) or privately-owned (8%), which leads to using a Build Operate Transfer (BOT) form of project delivery system [45]. A third-party construction project management company is hired for 67% of the projects.
As per the financing mechanism, 74% of the tunnel projects result to be totally funded with public sources of funding, while remaining projects are developed under a project financing mechanism so that the SPV is responsible for providing, in part or in total, the sources of funding. These come under the forms of both equity and debt financing [46].
When it comes to the payment scheme, in approximately 70% of projects, the contractor is paid a lump-sum fixed price, for 27% of projects, construction contractors are reimbursed based on a unit price scheme (such as price per km of excavation, etc.), and only remaining 3% has a cost-plus fixed fee payment contract [47].
Finally, as far as the O&M service contract is concerned, 20 tunnels are directly operated by the government and associated governmental bodies, 6 are assigned a specific O&M service contract, and the remaining 13 projects are operated and maintained by the same SPV, which is also responsible for the design and build phases.
3. EXPLANATORY ANALYSIS AND INTERPRETA- TION OF RESULTS
Following the approach for the explanatory analysis introduced above, the results of the standard ANOVA, Mann-Whitney, Kruskal-Wallis tests and linear regression analysis are presented and discussed to infer the expected cause of the project, technical and governance variables on schedule delay or cost overrun.
3.1. Influence of Project Characteristics
3.1.1. Influence of Type of Facility
First, the type of facility, namely rail, road, mixed use, or metro tunnel, does not prove any significant relation with the project schedule and cost poor performance. However, direct comparison of rail tunnel projects versus all other types of infrastructures as a group shows a little significant difference in schedule delay and can explain a likely, tough weak, impact on the schedule delay of the sample projects (Table 3).
In particular, rail tunnels have a shorter schedule delay than other facilities. However, this result may reflect the definition of schedule performance itself, referred to here as the ratio of schedule variance over the original duration, so that a longer duration leads to a smaller percent schedule delay [48]. In fact, the average duration of rail tunnel projects is 8.6 years, compared to 6.4 years for road tunnels, 5.6 for mixed use, and just 4.75 for metro lines.
3.1.2. Influence of Region
The influence of the geographical region is not statistically significant, as shown in Table 4. The analysis focuses just on Europe and Asia, as the number of observations for the other regions is not enough to prove significant. This result is in line with the studies by Flyvbjerg et al. [21, 22], who state that the cost escalation phenomenon has a rather global diffusion, regardless of the region where the infrastructure is built.
A similar non-significant correlation is also found when it comes to analyzing national tunnel projects versus transna- tional projects, such as alpine base tunnels. This indicates that the involvement of multiple governments is not likely to be a predictor of cost and schedule poor performance.
3.1.3. Trends Over Time
In their studies, Flyvbjerg et al. [21], could not detect any cost and schedule performance improvements in the history. However, in recent years, construction technology, as well as project monitoring and control tools, have considerably evolved so that one would expect a possible learning curve effect on schedule and cost performance.
Performance Variables |
Number of Rail Projects |
Rail Mean |
Rail St. Dev. |
Other Projects Number |
Other Mean |
Other St. Dev. |
Significance |
---|---|---|---|---|---|---|---|
Schedule delay | 13 | 0.242 | 0.258 | 13 | 0.432 | 0.305 | 0.098 |
Cost overrun | 8 | 0.379 | 0.405 | 18 | 0.222 | 0.263 | 0.246 |
Performance Variables | Europe n | Europe Mean |
Europe St. Dev. |
Asia n |
Asia Mean |
Asia St. Dev. |
Significance |
---|---|---|---|---|---|---|---|
Schedule delay | 13 | 0.370 | 0.327 | 10 | 0.272 | 0.245 | 0.438 |
Cost overrun | 15 | 0.317 | 0.327 | 6 | 0.132 | 0.123 | 0.19 |
To understand such project performance trends over time, the sample projects are classified into three clusters according to their start date, referred to as the date the project is formally approved/authorized by the government, namely: those started before the year 2000, those initiated from 2000 to 2010, and those started after year 2010. The homogeneity of distributions for both schedule delay and cost overrun are measured for both categories using a Kruskal-Wallis test. Results do not prove significant.
However, a significant linear relationship between the project startup year and cost overrun is observed, as shown in Fig. (1): this means that recent projects have been gaining cost performance improvements. As one would think, the high R2 indicates that the project start date is probably not the only variable that can explain the cost overrun improvement trend (Table 5): the concurrence of other factors is likely to influence the improved cost performance of recent projects, such as, for example, refined project monitoring and control systems.
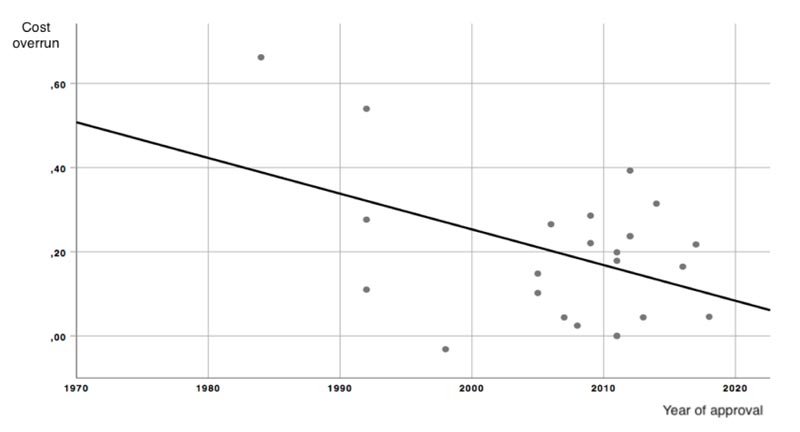
Model Summary | Parameter Estimation | ||||||
---|---|---|---|---|---|---|---|
Equation | R2 | F | df1 | df2 | Sign | Cost | b1 |
Linear | 0,2 | 5,496 | 1 | 22 | 0,028 | 17,219 | -0,008 |
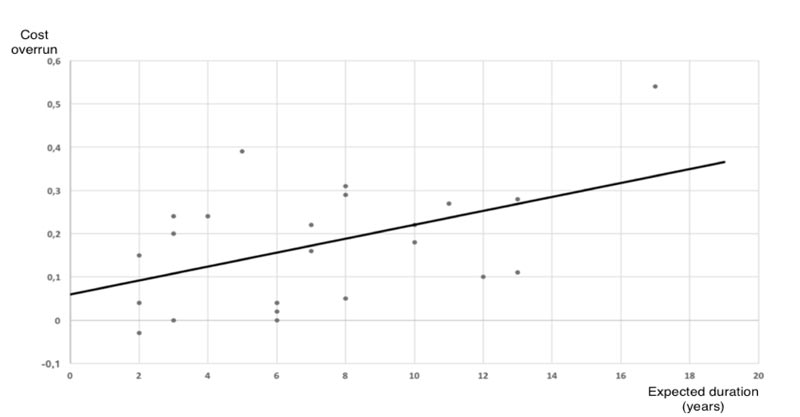
Model Summary | Parameter Estimation | ||||||
---|---|---|---|---|---|---|---|
Equation | R2 | F | df1 | df2 | Sign | Cost | b1 |
Linear | 0,24 | 6,48 | 1 | 21 | 0,019 | 0,06 | 0,02 |
3.1.4. Influence of Estimated Project Duration and Budget
The estimated project duration is also likely to impact on project cost overruns. Intuitively, it is harder to estimate long-term than short-term project budgets. This hypothesis is confirmed by the significant linear relationship between the expected duration as the independent variable, expressed in the number of years, and the cost overrun as the dependent variable, in percent, as shown in Fig. (2) and Table 6. This implies that the project schedule (work progress) is a determinant variable of future cost utilization behavior. This finding confirms the project duration, budget, and scope relationship which is regarded as the conventional elements of the project management triple constraints.
On the contrary, despite intuition that a larger budget could lead to lower project performance, the project budgeted cost does not show any statistically significant impact on schedule delay and cost overrun. However, the cluster of those megaprojects with budgeted cost greater than €4 billions have higher average cost overrun than smaller megaprojects.
3.1.5. Influence of Technical Variables
Some of the technical characteristics identified in Table 2 prove to have a significant relationship with a cost overrun, namely the tunnel length, the total length of tunnel tubes, and the number of cross passage tubes as given in Table 7, which reports the main parameters of linear regression analyses. These results are quite in line with statistical results by Flyberg et al. [22], who proved that the size of the scope of work is a likely determinant of cost overrun in large projects. Also, these results reaffirm the above mentioned studies on tunnel projects by Han et al. [38] and Hilar and Srb [39] who report that dimensional characteristics act as significant cost escalating factors.
The technical variables that are significant in this study are inherent to the intrinsic characteristics of project location and scope, which may be hard to manage and control by project managers and their project teams during the project execution stages. However, such characteristics can be considered during the feasibility and design phases when some flexibility in design is still available to make decisions about the possible reduction of the length of the tunnel, number of tubes, or at least, the number of cross-passage tubes. In other terms, appropriate design can reduce the size and complexity of the infrastructure as a likely precursor of future better cost and schedule performance.
Similarly, Table 8 reports the main parameters of linear regression analyses and proves no significance of the main technical variables on schedule delay, except the Infrastructure length. It can be interpreted that schedule slippages are experienced due to interconnections with the overall facility that includes the mega-tunnel as a part.
Variable | R2 | Significance | Constant | Slope |
---|---|---|---|---|
Infrastructure length | 0.016 | 0.655 | 0.223 | -0.001 |
Tunnel length | 0.393 | 0.001 | 0.044 | 0.007 |
Total length of tunnel tubes | 0.376 | 0.002 | 0.077 | 0.002 |
Internal diameter of single tube | 0.041 | 0.403 | 0.067 | 0.015 |
Distance between tubes | 0.070 | 0.382 | 0.094 | 0.003 |
Maximum altitude | 0.002 | 0.855 | 0.191 | 0.000 |
Maximum slope | 0.034 | 0.475 | 0.157 | 0.002 |
Number of cross passage tubes | 0.517 | 0.002 | 0.045 | 0.002 |
Distance b/w cross passages | 0.047 | 0.437 | 0.164 | 0.000 |
TBM excavation portion | 0.001 | 0.916 | 0.186 | 0.014 |
Number of TMB used | 0.009 | 0.749 | 0.180 | 0.004 |
TBM length | 0.712 | 0.001 | -0.042 | 0.001 |
TBM weight | 0.151 | 0.268 | 0.083 | 0.000 |
TBM diameter | 0.039 | 0.515 | 0.036 | 0.017 |
TBM average speed | 0.425 | 0.016 | 0.048 | 0.008 |
Traditional excavation speed | 0.036 | 0.760 | 0.344 | -0.32 |
Excavated rock volume | 0.000 | 0.994 | 0.195 | 0.000 |
Excavated rock weight | 0.893 | 0.000 | -0.017 | 0.012 |
Variable | R2 | Significance | Constant | Slope |
---|---|---|---|---|
Infrastructure length | 0,399 | 0,007 | 0,584 | -0,005 |
Tunnel length | 0,095 | 0,126 | 0,455 | -0,005 |
Total length of tunnel tubes | 0,062 | 0,251 | 0,415 | -0,001 |
Internal diameter of single tube | 0,030 | 0,453 | 0,526 | -0,023 |
Distance between tubes | 0,054 | 0,386 | 0,491 | -0,005 |
Maximum altitude | 0,011 | 0,637 | 0,319 | 0,000 |
Maximum slope | 0,024 | 0,540 | 0,282 | 0,003 |
Number of cross passage tubes | 0,062 | 0,303 | 0,429 | -0,001 |
Distance b/w cross passages | 0,068 | 0,297 | 0,402 | 0,000 |
TBM excavation portion | 0,096 | 0,197 | 0,167 | 0,262 |
Number of TMB used | 0,016 | 0,642 | 0,371 | -0,008 |
TBM length | 0,125 | 0,287 | 0,508 | -0,001 |
TBM weight | 0,002 | 0,890 | 0,314 | 0,000 |
TBM diameter | 0,005 | 0,797 | 0,434 | -0,010 |
TBM average speed | 0,001 | 0,912 | 0,349 | -0001 |
Traditional excavation speed | 0,516 | 0,172 | 1,316 | -0,210 |
Excavated rock volume | 0,117 | 0,303 | 0,443 | -0,016 |
Excavated rock weight | 0,243 | 0,600 | 0,431 | -0,005 |
3.2. Influence of Governance Variables
3.2.1. Influence of Ownership
Despite theoretical expectations, the variables pertinent to the governance model do not result as predictors of cost overrun and schedule delay in this study. In particular, project performance is not likely to be affected if the project is either managed by a governmental body, a public-owned project company, or a privately-financed SPV.
3.2.2. Influence of Financing Mechanism
Another influencing factor on project performance may be the funding system. However, also the differences, on average, between projects entirely funded by the public sector and privately-financed forms of Public-Private Partnerships (PPP) projects do not appear to be significant to explain potential cost overrun or schedule delay, as given in Table 9.
3.2.3. Influence of Contracting Mechanism
Another factor is the contracting mechanism between the promoter and the contractor: differences between the mean values of separated design versus integrated design contract categories, namely traditional Design-Bid-Build (DBB) infras- tructure procurement process versus integrated Design-Build (DB) contracts, prove to have statistical significance on cost overrun, while no influence on the schedule delay is observed, as shown in Table 10. This can be explained as follows. In a traditional separate DBB delivery system, any design change imposes heavy additional charges on the promoter that can lead to the substantial scope and budget creeping. Conversely, in an integrated DB or Engineering Procurement Construction (EPC) contract arrangement, the final cost increase is likely to be reduced as the cost estimates are made by the contractor [49].
Performance Variables |
Public n |
Public Mean |
Public St. Dev. |
PPP n |
PPP Mean |
PPP St. Dev. |
Significance |
---|---|---|---|---|---|---|---|
Schedule delay | 20 | 0.333 | 0.308 | 6 | 0.350 | 0.263 | 0.904 |
Cost overrun | 18 | 0.264 | 0.294 | 8 | 0.284 | 0.376 | 0.885 |
Performance Variables |
Separate Design n |
Separate Design Mean |
Separate Design St. Dev. |
Integrated Design n |
Integrated Design Mean |
Integrated Design St. Dev. |
Significance |
---|---|---|---|---|---|---|---|
Schedule delay | 9 | 0.304 | 0.284 | 12 | 0.420 | 0.304 | 0.387 |
Cost overrun | 10 | 0.364 | 0.361 | 12 | 0.153 | 0.186 | 0.091 |
Performance Variables |
Same Entity n |
Same Entity Mean |
Same Entity St. Dev. |
Different Entity n |
Different Entity Mean |
Different Entity St. Dev. |
Significance |
---|---|---|---|---|---|---|---|
Schedule delay | 15 | 0.340 | 0.280 | 9 | 0.410 | 0.300 | 0.545 |
Cost overrun | 16 | 0.290 | 0.320 | 10 | 0.240 | 0.320 | 0.698 |
3.2.4. Influence of O&M Factors
Finally, we investigate here the possible implications of O&M practices on project cost and schedule performance. The expected model is that the O&M duty assigned to the same business entity that is involved in the development period can act as an incentive to finish on time and within the budgeted cost so as to maximize the project's financial profitability. However, Table 11 shows that average differences between situations when O&M is given to the same business entity and those when this is given to another different service provider are not likely to influence neither the cost or schedule performance.
Overall, in this study, the governance variables show very little or no significant relationship with schedule delay and cost overrun. However, caution should be placed with this regard as the government data analysis is conducted at a high level using just a reduced number of tunnel mega-projects being executed in different social, legal, political, and economic environments.
Overall, a limitation of this study is related to the reduced number of tunnel megaprojects that are considered based on both the stage-one secondary-hand and direct survey data collection processes.
4. DISCUSSION, IMPLICATIONS AND FUTURE RESEARCH
This study is a theoretical contribution to better understand the main factors that may influence the chronical cost overrun and schedule delays of tunnel megaprojects. A dataset specifically focused on tunnel transport megaprojects did not exist and this paper makes it now available for future research and exploration by scholars in the tunnel engineering and construction fields.
Firstly, it provides data evidence about the poor cost and schedule performance of tunnel transport megaprojects, thus reaffirming the typical problem experienced by megaprojects in general [2]. Secondly, this dataset aims at integrating both technical and management data with the purpose of demonstrating that project performance is the likely result of a combination of proper engineering and managerial decisions, such as the choice of an appropriate delivery system [11]. Also, this paper gives an understanding of the main factors that may influence cost overrun and schedule delay of tunnel megaprojects in transport infrastructures. In particular, the analysis shows that project and technical complexity is likely to cause low schedule and cost performance in tunnel transport megaprojects.
Since the length of the infrastructure and similar technical aspects are often constrained by intrinsic morphology and norms, it becomes essential to improve project delivery via using integrated Design-Build contract mechanism, such as EPC or BOT, as well as adopting associated suitable project estimating, monitoring and control methodologies and tools to pursue improved time, cost and quality performance goals [50, 8]. From the point of view of the project governance, although not statistically significant, the establishment of a project company or SPV seems to improve the project cost and schedule performance than a direct management by the government.
Overall, the analysis suggests that the variables explaining various aspects of complexity play a role in cost and schedule deviations [11, 13]. These results are aligned with previous studies about the factors that explain the poor schedule and cost performance of megaprojects, in general. In particular, these reaffirm that a combination of technical and governance characteristics determine the cost performance, while the schedule performance is likely to be driven by the technical characteristics pertinent to the complexity of the infrastructure.
Future research is directed towards extending this empirical analysis to smaller tunnel construction projects in order to figure out if the size could also be a determinant of project performance. In other words, it would be important to explore whether the size and complexity of the tunnel infrastructure are a peculiar factor of low performance for megaprojects only, which is a main theoretical assumption and conclusion of this study, or it indifferently applies to all tunnel sizes. So far, one can conclude that tunnel megaprojects lag behind schedule and experience overrun cost because of their complexity and large size. However, future research may conclude that complexity is the intrinsic nature of mega-tunnels.
This study is a confirmation of what has been recently argued by Turner and Xue [51]: “as megaprojects are usually complex, so small changes in input can lead to disproportionate changes in output. So the time and cost targets at the start can have little validity. They are useful targets, not values which can be used to judge success or failure”.
CONCLUSION
This paper presents an empirical analysis based on a dataset collected via reports and first-hand questionnaires submitted to the project promoters and sponsors of 39 tunnel construction megaprojects internationally. It examines the statistical influence that the main project, technical and governance factors could have on cost and schedule performance. Analyses reveal that the most significant factors of cost overrun are those related to the project complexity, namely: the length of the tunnel, the total length of the tunnel tubes, the number of cross-passage tubes, and the type of project delivery system. The most significant variable which impacts the project duration is the length of tunnel infrastructure. This study reveals that the size and technical complexity may be crucial variables to explain the cost underperformances that are typically experienced in tunnel transport megaprojects. Also, using the traditional Design-Bid-Build delivery system may result in greater cost overrun compared to using an integrated design-build system.
The results are proposed to both scholars and project managers as an attempt to help improve the performance of future tunneling transport megaprojects and making better technical and managerial decisions at the feasibility and early design stages. The study offers a practical implication and lessons learned for decision-makers, public managers, project managers and designers who will be involved in the future construction of tunnel transport megaprojects. In particular, it is shown that one of the main drivers of cost overrun and schedule delay is the complexity of the infrastructure, including the length of the tunnel, the total length of tunnel tubes, the number of cross-passage tubes, and the delivery system. The importance of project complexity in driving the performance of megaprojects is not necessarily new knowledge, but the analyses conducted in this study help to quantify and validate this important impact factor for tunnel megaprojects.
CONSENT FOR PUBLICATION
The contributions of all participants were voluntary and we have therefore obtained their consent.
AVAILABILITY OF DATA AND MATERIALS
The data supporting the findings of the article is available within this article and Archived Datasets at https://www. dropbox.com/sh/4ftjghea2tq0h1u/AADzZNfUpYdgNiD58 dq9InA0a?dl=0”
FUNDING
None.
CONFLICT OF INTEREST
The author declares no conflict of interest, financial or otherwise.
ACKNOWLEDGEMENTS
The authors are grateful to TELT Tunnel Euralpin Lyon Turin, public promoter of the alpine base tunnel from Turin to Lyon, for originating the need for this benchmarking research, establishing cooperation with Politecnico di Torino, and providing active collaboration and access to the network of tunnel operators worldwide who were interviewed for data collection. The authors also wish to acknowledge Ms. Tea Turitto, a former graduate student at Politecnico di Torino, for her contribution in developing this research and preparation of the article.